CONCLUSIONS & RECOMMENDATIONS - CENTRIFUGAL COMPRESSORS
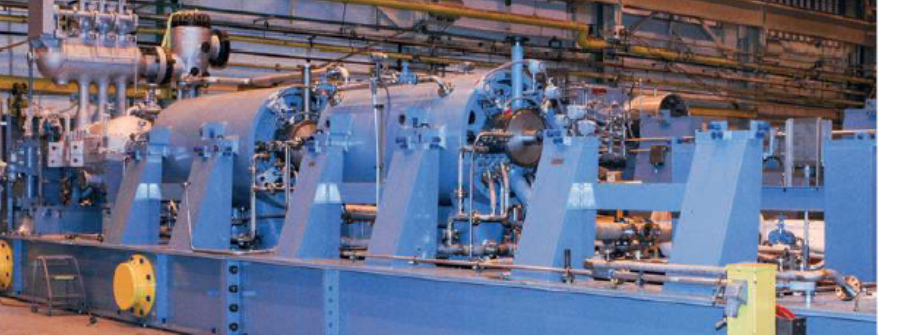
To obtain high-quality conclusions and recommendations for turbomachinery, the following technical actions can be applied:
- Data collection and analysis: Collecting and analyzing relevant data is critical to making informed conclusions and recommendations. This involves using a variety of measurement tools and techniques to gather data on parameters such as temperature, vibration, and performance. Advanced data analysis techniques such as machine learning can be used to identify patterns and correlations in the data.
- Root cause analysis: Identifying the root cause of any issues or problems with the turbomachinery is essential to making effective recommendations. This involves using techniques such as fault tree analysis, failure mode and effects analysis, and other methods to identify the underlying causes of any issues.
- Testing and validation: Before making any recommendations, it is important to test and validate any proposed solutions. This can involve testing individual components, conducting simulations, or using other techniques to ensure that the proposed solutions will work effectively.
- Continuous monitoring: After any recommended solutions have been implemented, it is important to continuously monitor the performance of the turbomachinery to ensure that the solutions are effective and that there are no further issues. This can involve using sensors, monitoring software, and other tools to track performance over time.
- Documentation and reporting: Proper documentation and reporting are essential to ensure that conclusions and recommendations are communicated effectively and that all stakeholders are aware of any recommended actions. This involves creating clear and concise reports, providing detailed technical documentation, and communicating with stakeholders to ensure that everyone is informed and on the same page.
Overall, applying these technical actions with high-quality control can help to ensure that conclusions and recommendations related to turbomachinery are accurate, effective, and reliable. It requires a deep understanding of the machine’s design and operation, as well as specialized knowledge and expertise in data analysis, root cause analysis, testing and validation, and documentation and reporting.
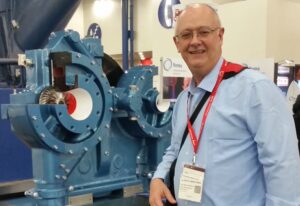